Fake Bananas
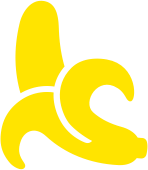
Fake Bananas is a fake news detector web app based on stance detection, natural language processing and machine learning.
At HackMIT 2017, Fake Bananas finished in the top 10 teams out of over 400 teams and 1250 hackers. Fake Bananas also
won Best AI/Hack for Social Good from Baidu and the prize for the Most Interesting Use of Data from Hudson River Trading.